Audio Super Resolution Turns Low-Quality Voice Samples into High-Quality Materials
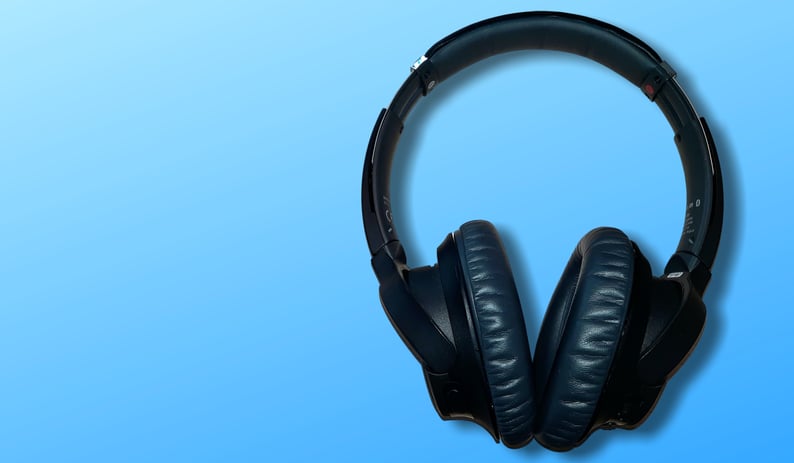
Machines turning text into speech is nothing new. Professor Stephen Hawking communicated with the world using a computerized voice for decades. Thanks to gen AI-powered neural networks, the quality of synthesized speech is improving. According to the latest data, the speech and voice recognition market is on the rise and is projected to reach $26.8 billion by 2025.
One of the most common problems in voice cloning and recognition is converting poor-quality recordings. For the longest time, it was nearly impossible to recreate someone’s voice using low-quality Skype calls or zoom recordings, mp3s, or other older voice samples. But with Respeecher’s super resolution algorithm, AI speech synthesis can now recreate voices without high-res recordings.
What is audio super resolution?
Speech technologies have been in development for decades, from the more common signal processing to modern voice synthesis. The last decade has seen a beacon of progress due to new artificial intelligence paradigms. In 2022, Respeecher is able to recreate the voices of anyone regardless of the source audio recording’s quality thanks to the powerful audio super resolution, resulting in a realistic AI voice.
With the emergence of gen AI, audio super resolution has become a reality, and is somewhat similar to image super resolution that can enhance the quality of images. Deep learning algorithms are used to turn low-quality images into high-res across a wide spectrum of industries, from medical to media and even surveillance.
Deep learning techniques have successfully solved the problem of video and image super resolution. Today, deep learning algorithms are able to enhance the quality of audio recordings.
With a low-quality recording, voice AI technology cannot precisely recreate speech and accurately mimic the pitch, tone, and pace of a real human voice. For example, if you wanted to generate a synthetic voice, you would need to not only upload a script but also an audio recording of the voice you intend to recreate. In some cases, the audio quality isn’t sufficient. That’s why Respeecher has developed the super resolution algorithm for clients without high-res sources.
Until now, the only method at our disposal was via our internal tool used by our sound designers and editors. But as more and more people came to us wondering if we could help with enhancing the audio quality of recordings, we decided a standalone product was needed.
Any old audio tapes, compressed audio files, or FaceTime recordings are now recoverable and can be turned into high-resolution audio with Respeecher's AI speech synthesis technology, powered by its super resolution algorithm.
Learn more about audio super resolution in our whitepaper.
Audio super resolution at Respeecher
To clone a voice, technicians need to feed audio recordings of the source speaker into a deep learning neural network like Respeecher AI speech generator, powered by voice AI. The neural network then identifies patterns in that voice like tone, speed, stress, rhythm, and pronunciation to create a voice model that can voice entirely new scripts.
Thanks to audio super resolution, Respeecher managed to revive the memorable voice of Manuel Rivera Morales. It was one of the most complex data targets we’ve ever worked with as all the recordings were made in the 70s and 80s. The level of quality was due to:
- Many instances of background noise that needed to be filtered out
- 16 kHz sampling rate
- Low-fi microphones that couldn’t catch every sound
- Quality and information losses due to the data transfer from analog to digital format
Thanks to our skilled technicians, voice editors, and sound designers, Respeecher faced these challenges head-on and succeeded with flying colors. Our team managed to synthesize Morales’ voice for the entire broadcast, clocking in at five hours. It took us 10 days to train the AI neural network and five hours to synthesize the speech. Even Rivera Morales’ daughter was speechless when she heard her father’s voice commentating the Puerto Rican National Women’s Basketball Team’s debut match against China at the Olympic Games. Our realistic AI voice technology made it possible to bring his voice back to life with astonishing accuracy.
Sample of the AI generated voice of Manuel Rivera Morales used for voice synthesis:
Sample of the AI generated voice Manuel Rivera Morales voice commentating the match in 2022:
The technologies behind audio super resolution
Respeecher’s audio super resolution network is a GAN-based neural audio enhancer that fills the gaps of missed bandwidth and adds extra resolution. The audio enhancement is performed by a well-trained neural network that scans the frequency range of the input audio, identifies any missing points, completes the audio spectrum, and generates a high frequency that is seamlessly blended with the original audio. Based on high-resolution audio samples, the network predicts signals that the input audio misses.
Nowadays, Respeecher can enhance audio recordings from 16.05 kHz to 44.1 kHz, which are more than enough to add air and brightness to the listener's experience. We’re currently working on new features like noise reduction and dereverberation, audio decompression, and bit-depth super resolution.
Final note
Although speech synthesis still has its own issues to overcome, we’ve managed to alleviate one of the biggest obstacles in recreating speech — Respeecher can now enhance low-quality audio and fill in the missing bandwidth gaps. Contact us if you want to learn more about Respeecher’s latest breakthrough in AI voice synthesis.

- AI voice synthesis
- artificial intelligence (AI)
- speech synthesis
- Manuel Rivera Morales
- AI voice
- AI deepfake voice
- Voices
- Respeecher
- Respeecher’s voice cloning algorithms
- audio super resolution
- audio
- Respeecher super resolution algorithm
- AI speech synthesis
- AI generated voice
- Respeecher for Business
- AI speech generator
- voice ai
- gen AI
- realistic AI voice